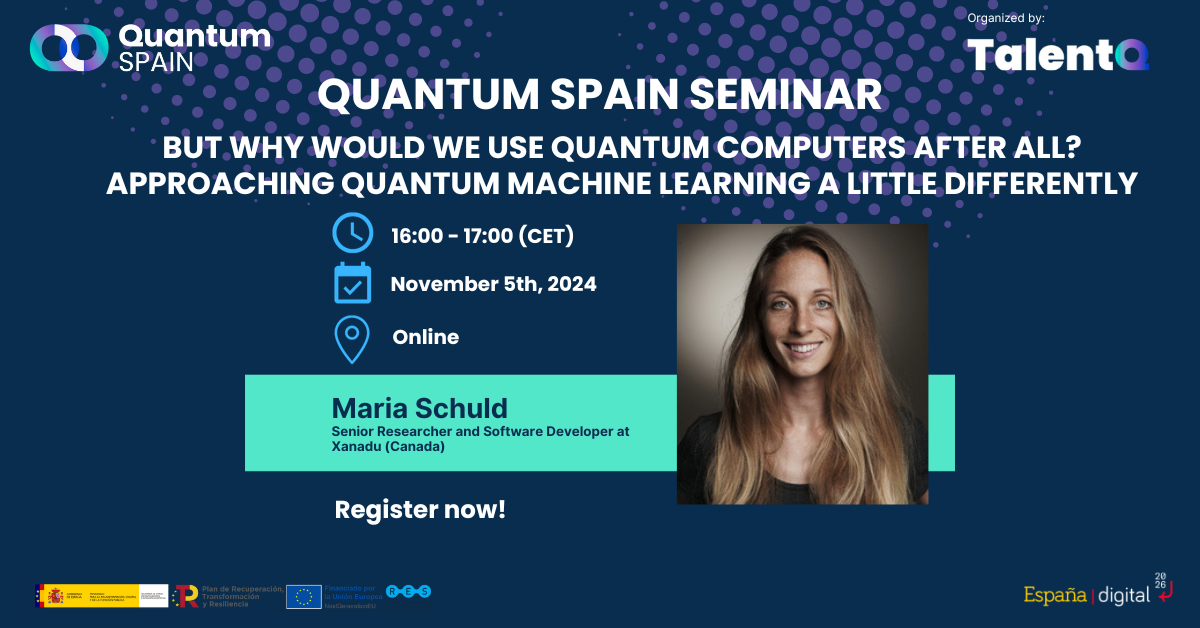
Title: But why would we use quantum computers after all? Approaching Quantum Machine Learning a little differently
Abstract: The last years of research in quantum machine learning have taught us a lot. There are problems where quantum computers have a provable advantage for learning (just apply Shor somewhere!). Training variational «quantum neural networks» is a matter of a few lines of code, but you need to be careful not to be dequantized, and the results are a little disappointing. We all hope that things look better for «quantum data». And a lot has been written about barren plateaus. But why, on earth, should we use quantum computers for machine learning at all? It seems that we have not come any closer to answering this question. In this informal talk based on arXiv2409.00172, I suggest a slightly different approach to QML: One where we stare hard at a famous family of quantum algorithms, try to understand why they work (not when they are faster) and muse how this could be turned into a learning principle. Expect no speedup and no end-to-end learning algorithm, but a lot of educated speculation.
Registration: https://events.teams.microsoft.com/event/d34819e1-3e2b-411d-87dc-a98bd8607793@8f0d452c-b7a4-4964-b810-8c397374477b